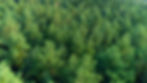
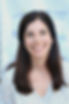
Maya Almagor
Department of Biomedical engineering,
Technion - Israel Institute of Technology,
Haifa, Israel
Research Overview:
Spectral Imaging for Digital Pathology and Cancer Classification using Image Processing and AI Tools
A Bit About Me...
Education
2018-2022
2022-2025
2022-Present
B.Sc., Biomedical Engineering, Technion – Israel institute of Technology
M.Sc., Biomedical Engineering, Technion – Israel institute of Technology
Physics and Algorithms Engineer, Medtronic
Conferences
June 2022
December 2022
May 2023
April 2024
Annual Biomedical Engineering Faculty Posters Day, Technion -
First place award for best poster and presentation
The first Technion Biophysics retreat
ISM Microscopy Conference - poster presentation
BME Annual Research Day, Technion -
First place award among MSc. students
My Research
Keywords: Digital Pathology, Spectral Imaging, Cancer Detection and Classification, AI in Biomedical Imaging
​
Rapid Spectral Imaging (SI) is a new method that allows us to acquire the full visible spectrum for every pixel of a measured object. In my work, I am developing methods for using spectral imaging for cancer diagnostics and prognostics. Here I concentrate on one work where I focus on the development of AI-based methods of SI data for detecting thyroid cancer.Thyroid cancer is the most prevalent endocrine cancer, and its incidence has been steadily rising. It is typically diagnosed by pathologists through visual inspection of thyroid tumor resection specimens, a time-consuming and resource-demanding procedure that can lead to misclassifications with critical prognostic consequences.We present a highly accurate diagnostic system for thyroid cancer that leverages a newly developed optical system that rapidly measures the full visible light spectrum of each point on the tissue section. Measurements are acquired directly from routinely prepared hematoxylin and eosin-stained resection specimens, eliminating the need for additional procedures. The tissue samples used in this study were annotated by expert pathologists, and their spectral images were then analyzed through deep learning and machine learning algorithms. This integration of rapid spectral imaging with artificial intelligence enables robust differentiation between normal and tumor cells. By targeting diagnostically relevant features in standard stained tissue sections, this approach provides a straightforward yet powerful approach to the pathological assessment of thyroid cancer. This method can provide critical support for pathologists, facilitating accurate and timely evaluations of thyroid cancer cases and holds promise to diagnostics in other cancer types.